Customer Experience Insights by Marc Preusche
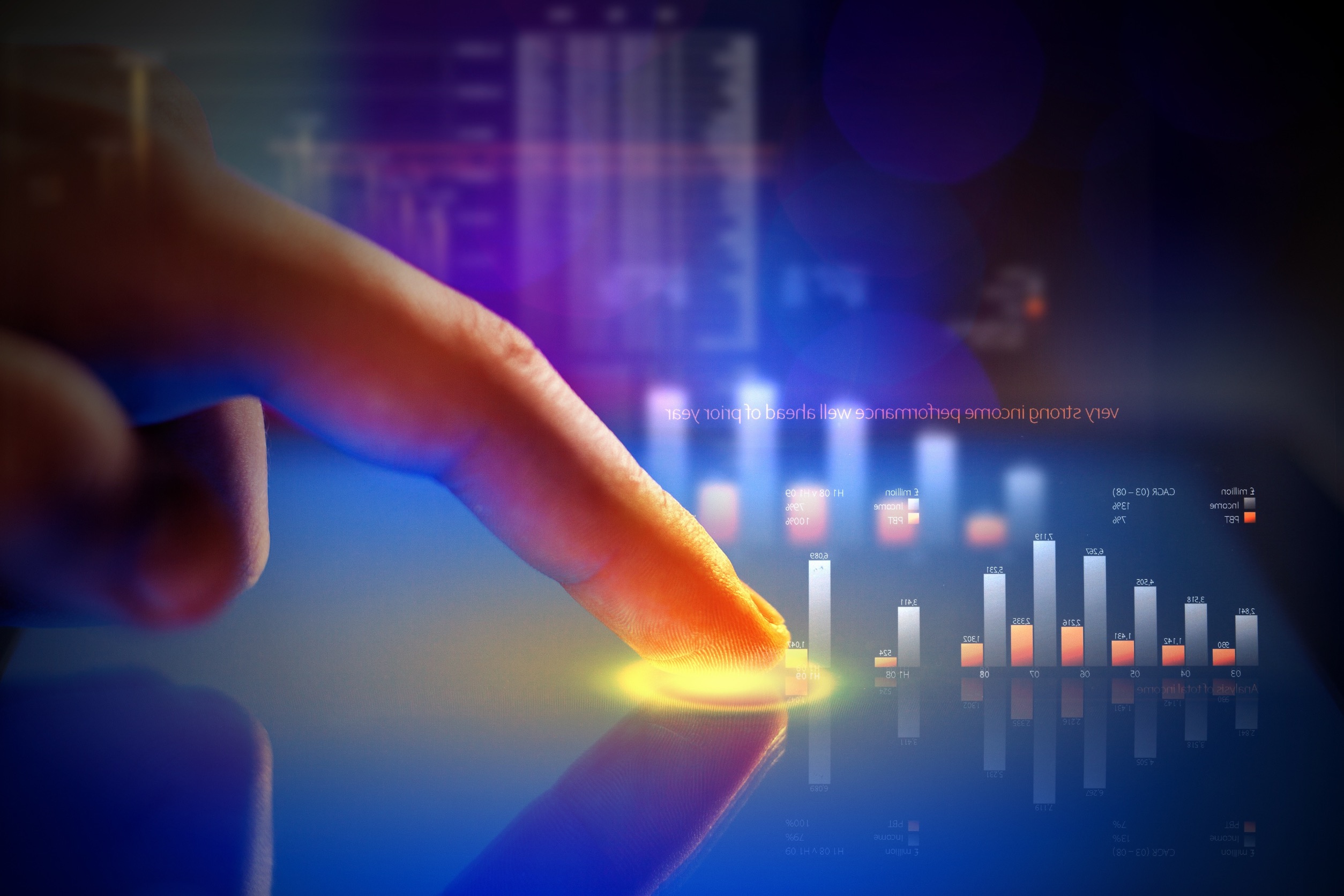
The following article is an excerpt from an interview with Marc Preusche, which we conducted as part of our work on our white paper on data-driven customer experience optimization. Marc is Managing Director of DEPT DATA & INTELLIGENCE (formerly LEROI Consulting). Among other things, current trends and strategies of customer experience (cx) optimization such as real-time personalization or augmented analytics are discussed in detail.
ODOSCOPE: Hello Marc, What is essential to be able to measure customer experience and subsequently optimize it?
Marc Preusche: I don't think it's really something technological in the beginning, but something cultural or organizational. There is an understanding within the organization.It is the awareness that customer experience is not only important, but essential for the success of a company.
As soon as this is achieved, you can slowly start to give the whole project a little more substance. I think one of the first tasks that needs to be tackled is to "cleanup" a bit. First forget the whole BI part, forget Marketing Intelligence.Clean up the standard tools: Clean up your web analytics, app analytics, CRM.
Then two things happen in these areas: on the one hand, a proper data quality and on the other - which is much more important from an organizational point of view -that what a proper data quality actually is is also perceived. This means that people really want to understand it and work with it. And then two things come together: On the one hand the perception: "Hey, we have good data, we can work with it! ", and on the other hand, the cultural thing I was talking about earlier.
The aim is to do a little more with the customer experience. Although "a little more"is not enough, it is more important to concentrate fully on the topics of user experience and customer experience as a company. These two aspects together then form more or less the basis and also the catalyst for the whole project to function.
ODOSCOPE: That's a very exciting perspective. Even since there is currently the opinion that one always needs complex tools and would have to spend large amounts of money in order to be able to work properly with the data. Is that right?
Marc Preusche: It always depends on the company.For some companies a simple Google Analytics is enough, others may also need the various user-centric tools. And then there are some who tick like a few Finnish companies with which I have worked together. I asked them: "Hey, what does your Analytics set-up look like?" and then they laughed at me and replied: "We just have a tracker, these are our logfiles, which we drilled out a bit. With us everybody can SQL, with us everybody can Python. We don't need this." I think that's cool too!
ODOSCOPE: Very cool anecdote! When does real-time optimization make sense in comparison to a BI alternative? So a classical "overnight calculation" - this is not so fast, but usually a larger data basis is offered. So where and when is real-time vs. machine learning or non-real-time recommended?
Marc Preusche: In my opinion, regardless of what you do, it must always be a kind of hybrid. Take, for example, the scenario where a completely new user you've never seen looks into your app. He downloaded it directly. You don't know where he's from. You didn't use app analytics like Adjust.Then what are you doing?
Then only a clean real-time editing will help you to record some information about the used phone types and the like. So it is not unimportant to have a certain component that works like a realtime optimization. This should still provide the necessary information about user preferences. Or also about when you should play a push message or when you should insert which onsite banner. This is difficult to say if you don't have a complete use case.
In any case, you should really have a look at this hybrid, which rather goes in this direction. But you should still do most of the work in the background, because then it is more controllable, at least at the beginning. Before you do a complete data-driven personalization, you always have to rely on a set of rules and usually don't use a real-time component.
With the passage of time, however, you come to a point where you can think: "What can I do with the real-time component at all? What added value does that provide?" And as soon as you have come to a decision and say that the real-time component has marginal added value compared to the non-real-time component, you have arrived at a point where you can have a look at the hybrid.
My favorite example from the area of real-time personalization is the recommendation engine. What you can do in this area in real time, if you have more information about the user... Imagine for example: You're a fishing tackle store. An interested visitor has placed three or four parts in the shopping cart and your system automatically recognizes: "Most customers then also buy the fifth part". You just offer him the part in addition. This is a neat way to install the realtime component at the beginning. From a technological point of view it becomes relatively difficult and expensive. This is then mostly real big data.
ODOSCOPE: This means that one can already see that this is rather something for very large companies and that small companies may lack the necessary data basis. Right?
Marc Preusche: : I think it depends on the tool that is used. Let's take the Gartner model with the phases descriptive diagnostic, predictive and prescriptive, which is more or less personalization. Many people think that these are individual steps that have to be implemented one after the other. But that's not true. If you have a good tool in the area of personalization that delivers the amount out-of-the-box, you can jump directly to the prescriptive part and use a certain real-time component there.
And such relatively simple things like a recommendation engine: If the recommendation engine works properly it's great. This is not an ingenious fully machine-driven real-time calculation, since certain real-time components vibrate along, which could still be exciting. Again it says: It depends on the tool you use.
ODOSCOPE: In your opinion, what are the most promising trends in the field of customer experience optimization?
Marc Preusche: I find the entire area of augmented analytics particularly exciting. I mean support that does not work in the plastic sense of analytics - i.e. preparing data minimally, putting it in a nice package and then handing it over to someone as a table or chart. That's cool, but as a person you still have to think a lot. You still need a certain basic understanding and especially for many creative workers this is relatively difficult to implement. Often this area of work functions quite differently. Imagine going to a data scientist and telling him to be creative for once. That's not his job. In the creative field, this may be the case when a data scientist suddenly demands a basic analytical understanding. That is and needs a well-coordinated division of labour.
But what if you are a small company, can't afford a great data scientist, but have an augmented analytics platform? This gives you certain insights that are comparable to a good data scientist, but no complex table is made from the raw data. Instead, as in machine learning, certain knowledge is drawn from the table and displayed. I think this possibility will make a difference: Everyone has access to the data. Anyone can work with it. The only problem is that not everyone is designed to extract useful information from an analytics platform, interpret important findings and thus optimize their work. It just doesn't work as easily as you might imagine.
Augmented analytics really delivers this part completely to the machine, extracts the most important findings from the data, with which you can then improve the performance of your own company very well. The topic of augmented analytics can be a huge boost especially for the part of the working population without a hard analytical understanding. If you can jump directly to the results, i.e. automate the good analyst, the data scientist, so to speak, then you achieve a much better scaling of the whole thing.
Even as a human being, you probably learn much better. I think this is very exciting, because any system can be optimized with it. This strategy can also be used, for example, to improve the interaction between man and machine when it comes to personalization. I mean strategies where the machine tells you which segments work much better in which step than possible others when you test personalization.
Reasons for this can then be certain differences between the clusters, as in cluster analysis, for example, where one cluster perhaps wants to be addressed more emotionally, the other more with features and another only from the price. So very simple examples. And if the machine tells you that and tells you why the split is like that, you've already won a lot.
ODOSCOPE: That's right! Could you please give some examples of augmented analytics applications?
Marc Preusche: There are some things you can do with augmented analytics. I think what still has a lot room for improvement in the whole CX area is to include external factors such as football matches, festivals and the weather. I find the weather example alone very exciting.
There are some voices that claim that it has been proven several times that something like this has no influence at all, but I see it quite differently. If you now consider that an augmented analytics software can in principle give you an accurate weather forecast for a certain area at a certain time, then people will either not go to the cinema or even more so they will go to the cinema because there is an air conditioner there.
You can then divide this into different segments and adapt the different campaigns accordingly. For this you need some creatives, which go in this direction. In good weather in Frankfurt you might need content with a lot of sun, sweating people, which you quickly search out from your own archive, rate and then publish. But there's a huge storm in Leipzig - of course you don't want to drive this campaign there. With geo-splitting you can then apply for an accident insurance for hail damage.
ODOSCOPE: Good use case for the insurance industry!
Marc Preusche: These are then situational factors that can be wonderfully integrated into the augmented analytics context. You can try to generate such a split between different regions based on weather, football matches or based on events that simply take the local people along and carry them along. If you then understand these insights, these differences, you also understand the context, which is always relevant. It's all about context - whether it's KPIs or measurements. In addition, the context is usually implicitly given, but one may not even be aware of it.
The conversion rate is roughly between the number of regular customers and the number of sales. If one of the two rises or falls, the statement of the percentage can be reversed. But these are exactly the things that can be optimized by augmented analytics, because the fast up to experimental construction of a context facilitates the analysis enormously. As a quite banal continuation of the Frankfurt/Leipzig example: What good is it to me if I spend three days researching something I could have played the day before yesterday? A machine will do it for you within 20 seconds - or faster.